Getting Your MSP Into Big Data Analytics
Data analytics are now a must-have service for many companies. Here's what MSPs need to know about the big data world today to deliver winning data analytics service to clients.
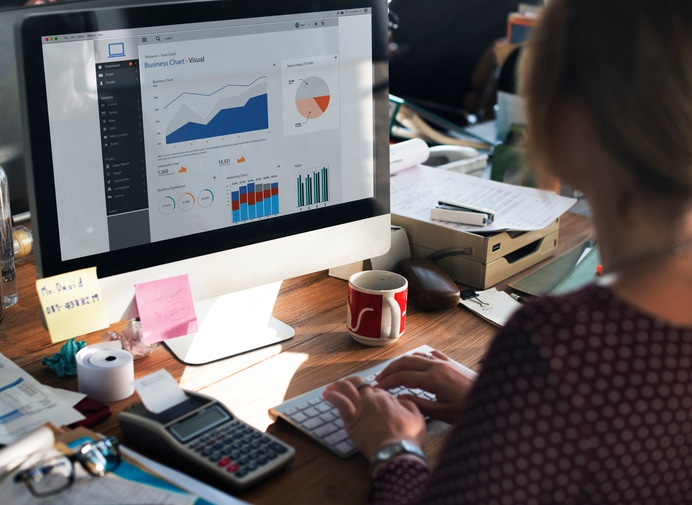
The big data world has evolved rapidly over the last decade. Ten years ago, only the biggest companies, like Google, used data analytics on a massive scale. They could do it because they built their own analytics platforms for big data, such as MapReduce, and they had massive infrastructures at their disposal.
Then, about five years ago, open source platforms for big data analytics began to appear, with Hadoop leading the way. This made it possible for many more organizations, including ones with more modest infrastructures, to leverage data analytics.
Data Analytics Today
Today, however, organizations are demanding more than just Hadoop. The newest trends in big data include:
In-memory analytics. In-memory analytics processes information that is stored in RAM, rather than on hard disks (the storage medium that Hadoop traditionally uses). Because RAM can read and write information much faster than disks, in-memory analytics delivers speedier results.
Real-time analytics (also called streaming analytics). It's no longer enough to analyze data a week, a day or even just an hour after it was produced. Today, companies want to be able to make data-based decisions in real time. For example, a bank might want to be able to detect and block fraudulent payment card activity using real-time data analytics before a payment transaction even completes.
How MSPs Can Deliver the Right Data Analytics Today
How can MSPs give their clients ultra-fast, real-time analytics? The answer is to take advantage of newer big data technologies tailored to solve the challenges outlined above. Those platforms include Apache Spark, which is made for in-memory analytics, and Apache Kudu, a platform for real-time analytics.
Other frameworks for building apps that use streaming data include deepstream. And companies like Syncsort develop solutions for streaming analytics on mainframe systems (which are a category unto themselves when it comes to big data, and beyond the scope of this article).
When it comes to actually implementing these platforms, MSPs should determine whether they have the in-house expertise required to deploy and manage the "raw" versions of platforms like Hadoop, Spark or Kudu themselves. These are all open source platforms, which can be downloaded and installed for free. But that requires a fair amount of know-how.
If an MSP lacks that expertise, it will get better value for itself and its clients by using a commercially supported distribution of big data platforms. In the interest of fairness, I won't name any particular vendors, but Wikipedia offers a list of companies that specialize in big data services, both on-premise and in the cloud. Many provide distributions of platforms like Hadoop and Spark.
All of the major public cloud providers also offer hosted implementations of the most popular data analytics platforms. Azure offers HDInsight; Amazon has EMR. These services are the easiest way for most organizations to use big data tools. MSPs could potentially leverage them as part of their offerings — although to do that, of course, an MSP would have to be careful to add value on top of the service that customers could get directly from the public cloud.
About the Author
You May Also Like