How MSPs Can Help Customers Harness AI and the Promise of Big Data
Customer needs dictate whether data lake or data pipeline is best for storing, analyzing data.
April 30, 2021
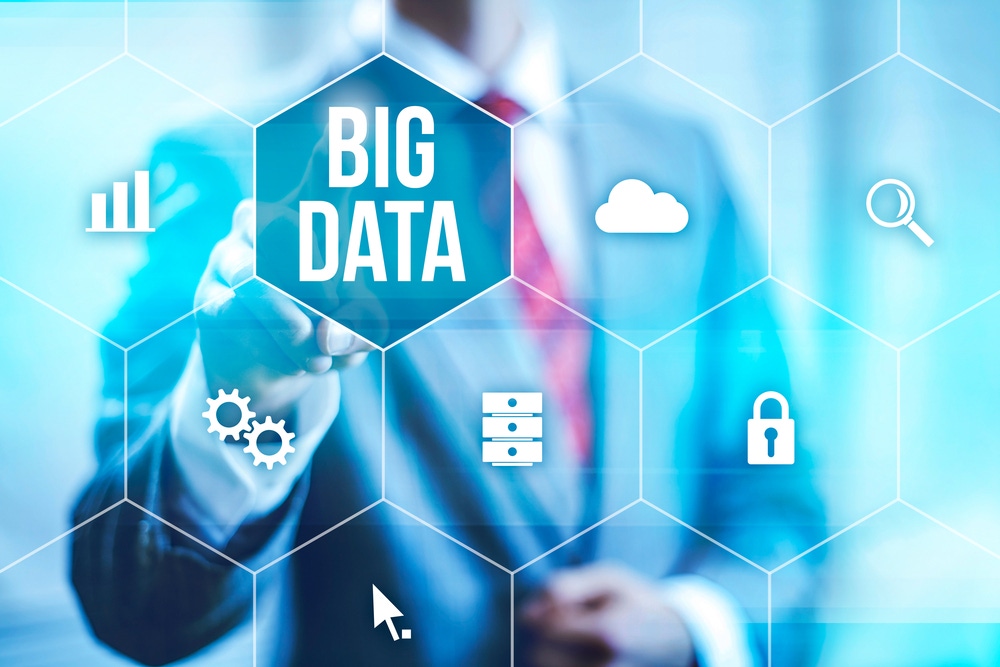
By Rich Itri
Rich Itri
Data is the fuel that drives the digital world and businesses are increasingly riding it to greater success. The more you know the further you’ll go, and thanks to the internet of things, it’s easier than ever to gather data. Then, with artificial intelligence and machine learning tools, you can unearth, create and capitalize on insights and opportunities that are just waiting to be discovered in this mountain of information.
Still, before all that data can be put to work, it must be stored and secured. Managed service providers can help their customers do so in the safest, most cost-effective way, while ensuring data is ready for AI and ML. Two popular methods for doing so are via data pipelines and data lakes.
Both can be useful for storing and analyzing data. However, the right approach depends upon customer needs, and if the MSP provides the wrong direction, a data lake or pipeline can quickly turn into a data swamp.
Learn the Difference
What’s the difference? As an MSP, there are a few key definitions to get under your belt to ensure you provide the right advice.
For starters, a data lake is a central place where a customer can store all data, regardless of scale. This includes structured data, which is formatted and highly organized so it’s easily searchable, and unstructured data, which has no format or organization and is much harder to collect, process and analyze.
Data lakes offer a wide spectrum of information to the user and work with many types of analytics, resulting in better, more refined data. Organizations who implement data lakes can really use just about any method of analytics, including ML, and can leverage information from additional sources, such as click-streams and social media.
A data pipeline, on the other hand, is part of a system that filters and formats data. This efficiently culls insights without involving superfluous information. The result is concise data that’s easier to report, analyze and put to use. Data pipelines can also produce details customized to fit a company’s needs by reducing data noise and focusing on criteria aimed at a specific goal, which in turn increases business intelligence efficiency.
How are they used? Both data lakes and data pipelines can support AI. However, each possesses unique features and benefits which can help identify which data solution will work best.
ML must trawl volumes of data to find useful trends that give information real meaning. This is also the reason data lakes are beneficial to AI. In fact, many of us encounter data lakes and ML, unknowingly, every time we use the facial recognition feature on a mobile device. And, as an example, the more you use facial recognition on your phone, the more easily it’ll recognize you even when wearing accessories like glasses or a hat.
Additional ML and data lake applications range from the refining of search engine results to virtual personal assistants to social media advertising services. Further, large data lakes enable people from …
… various functions to use the analytic tools they prefer in order to find information that best meets their specific needs.
Data pipelines typically serve as the backbone of AI embedded applications and are essential to running them. If you wanted to unlock your phone, and FaceID had to search through every detail in its memory for all images that remotely resembled your face, it wouldn’t be useful – you’d get frustrated waiting and type in the password. A data pipeline only draws upon relevant previous information; therefore, the process is much faster.
Then again, data pipelines do more than just reduce data volume; they can also draw upon data from additional sources, while eliminating duplicate and conflicting information.
Choose or Lose
So how do MSPs help customers make the right decision?
As a rule of thumb, if your customer’s goal requires a variety of data types from many sources – and they want the flexibility to creatively analyze data that’s been consolidated – a data lake is the way to go. But, if they know what type of information they’re looking for, and can use a reliable and constant stream of clean data, steer them to a data pipeline.
Big data isn’t going anywhere because it provides powerful insights that likely could have a significant impact on a company’s fortunes. Even so, regardless of the approach – data lakes or data pipelines – help them choose or they’ll lose a tremendous advantage and market share to savvier competitors.
Rich Itri is a senior vice president of professional services (CIO Advisory) at Eze Castle Integration. Rich has more than 22 years of IT executive experience, spending his career managing IT within the financial services industry. Previously, he was managing director and chief technology officer for PJT Partners, a boutique investment bank; principal and chief information officer for Sky Road; and chief information officer at both Arrowhawk Capital Partners and Arbalet Capital Partners. You may follow him on LinkedIn or @EzeCastleECI on Twitter.
Read more about:
MSPsYou May Also Like