The Next-Gen MSSP: Machine Learning at Scale
Machine learning-based cybersecurity solutions can deliver a competitive edge.
November 2, 2020
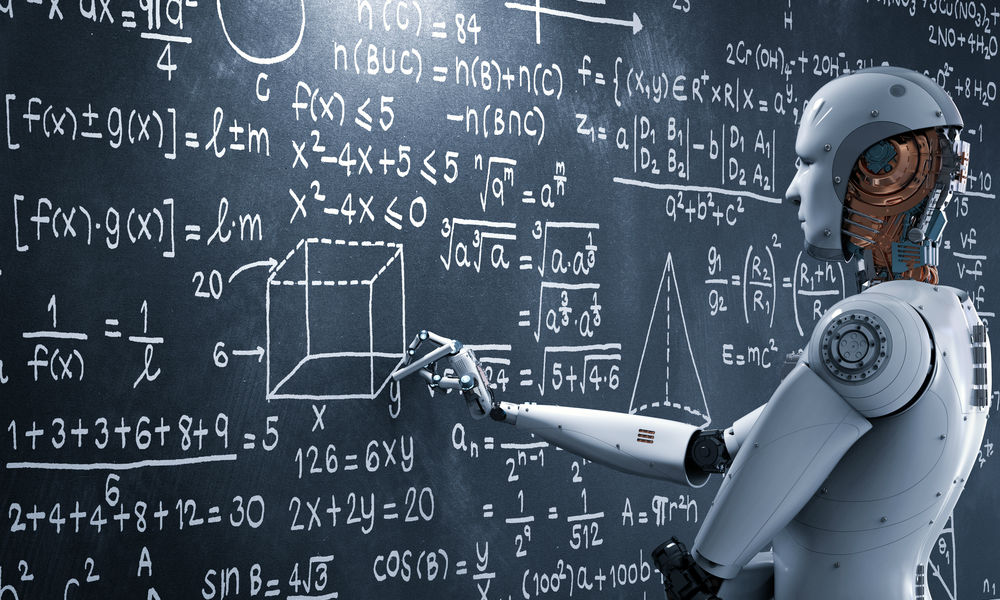
By Chris Triolo
The next-gen MSSP is upon us. MSSPs are competing for customers with an ever-growing number of cybersecurity solutions on the market. Providing a machine learning-based cybersecurity solution can give MSSPs the competitive advantage they need over traditional systems, enabling them to deliver maximum value and security to end-users.
The breakthrough concept behind successful machine learning security software lies in integrated reasoning. It’s the idea of correlating many points of view (models) to make better, more accurate decisions. Stitching together evidence is not something an analyst has time to do for all but a few incidents. Machine learning models, however, are perfect for the task.
Why Human Analysts Need Machine Learning
Analysts may be highly trained with years of experience, but they’re still only human. What that means is that their ability to assess all security alerts that come their way is limited. They’re also restricted by the limits of their memories, attention spans and awareness, and they bring human biases that can confuse investigations. Filtering is something the industry has come to accept as the solution to address this exponential problem of data growth and lack of skilled analysts, but what are you filtering out?
Machine learning (ML) is a term for computer models that improve automatically through feedback and experience. ML algorithms build a mathematical model based on training data to make predictions or decisions without being explicitly programmed to do so. When MSSPs add machine learning principles in their systems, those systems adapt over time, which gives those MSSPs an advantage against malicious actors.
An MSSP is constantly feeding large volumes of data into its tools. If these tools are operating based on a Bayesian reasoning system, it will allow these tools to improve and update their beliefs from experience, resulting in a smarter product. Bayesian reasoning relies on an interpretation of relative probabilities when circumstances are uncertain. Moreover, it is a system that applies machine learning and AI techniques to make a mathematical calculation to determine if an event or set of events is malicious and actionable – which is ideal for security operations.
ML Is Your Friend
Today’s advanced cybersecurity automation software uses Bayesian logic, along with other modeling approaches, to find the most likely solution to problems involving enormous volumes of data. Thus, it can consider near-infinite amounts of network telemetry data, user data, operating system data and threat intelligence. It can operate without bias; just because it’s seen a million instances of the same alert type that weren’t malicious, it’s not going to assume that this one isn’t.
It also doesn’t forget: a machine uses mathematical calculations along with its 180-day or more short-term memory to analyze streaming security events in real time. Its memory incorporates what has happened (or is happening) in the environment, down to the system or user level. It can ask a huge number of detailed questions in a short time span. This makes it good at …
… security monitoring, since it involves asking repeatedly, “Is this event strange? Is it related to other events that are occurring, or have occurred, elsewhere? Are vulnerable assets involved? Or known malware? Or sensitive data?”
What ML Provides the MSSP
Most MSSPs that perform security monitoring for their customers need to support a broad list of vendors and telemetries. Most use brand-name security information and event management (SIEM) tools in addition to relying on their own engineering to do data parsing and build custom integrations, management tools and basic automation. This usually requires time, money and people to maintain their systems, as well as launching services with new customers.
The reality is that security monitoring can now be done algorithmically with customers getting complete incidents in their UI. For any incident to be removed from their queue, they’ve got to provide feedback, and that becomes supervised machine learning. An MSSP can crowdsource the supervised machine learning across its entire customer base, which means everybody benefits. This even encourages turning up the sensors all the way and getting as much information as possible. More information equals better decisions.
Looking Ahead
The current MSSP security monitoring offering isn’t sustainable in the face of today’s massive alert volumes. Hours of work investigating false positives for security sensors or SIEMs still fails to identify all critical incidents. This is due to technology and human limitations. Which is where ML can be a big help. Supervised learning enhances security monitoring over time. More data sources will only increase that learning and sharpen the tools with which security analysts combat cyberattacks. MSSPs that leverage ML will deliver maximum value and security to end users, providing added value and establishing a key differentiator in the fight against cybercrime.
Chris Triolo is chief customer officer at Respond Software. His security experience includes stints as VP of professional services at ForeScout and global VP of professional services and support for HP Software Enterprise Security Products. He also worked at Northrop Grumman TASC supporting various Department of Defense and government customers. Follow him on LinkedIn.
Read more about:
MSPsYou May Also Like