Recognizing the Limitations of Big Data and Data AnalyticsRecognizing the Limitations of Big Data and Data Analytics
As big data use cases extend to realms like smart devices and driverless cards, data analytics can't always deliver the ultra-accurate results that they require.
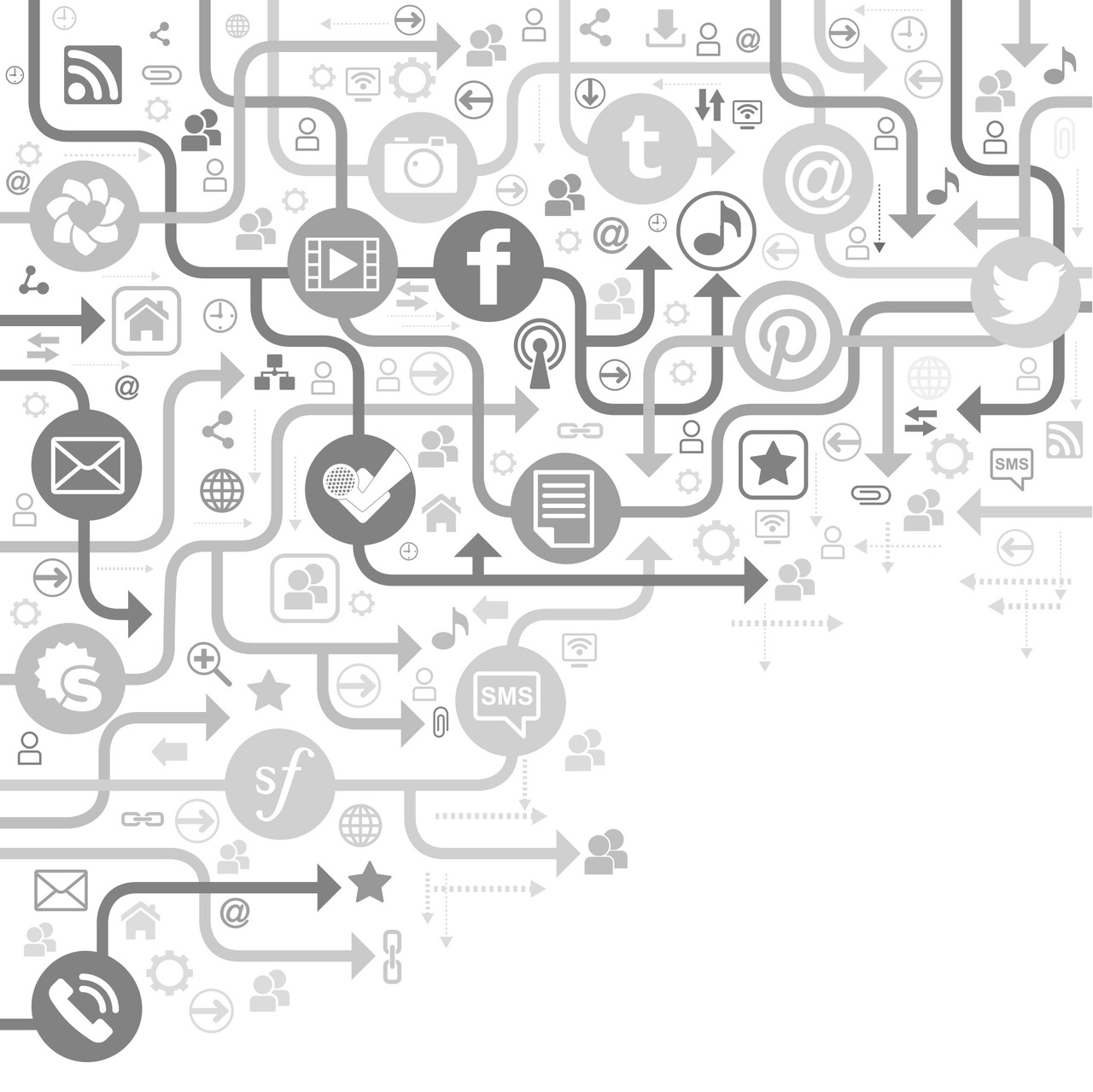
Is big data accurate? That depends not just on how you use big data, but what you use it for — and it’s a key question to weigh before deciding whether big data and predictive analytics can help or hurt you.
Big data and predictive analytics have become central to the way organizations large and small interact with users.
To a degree, that makes sense. If you want to scale your business operations beyond what you can handle using manual processes, you can take advantage of big data.
Using big data and predictive analytics, you can do everything from drive marketing campaigns to make software code write itself.
Predictive Analytics Challenges
Yet there is a major challenge surrounding big data and predictive analytics that can be easy for organizations to overlook.
The challenge is that there is a great deal of variability in what an organization hopes to get out of big data. The variability depends on the context of the big data use case.
In some situations, having predictive analytics results that are merely pretty good is more than enough to meet your goals.
In others, you need big data to drive insights that are nearly 100 percent accurate.
The latter results are very difficult to achieve. Even the best data scientists, equipped with the best big data platforms, can’t guarantee completely accurate analytics, no matter how much data they have to work with.
The Growing Big Data Problem
The big data use cases of the future call for highly accurate predictive analytics results. That’s a problem.
A few years ago, big data was used primarily for tasks like delivering product recommendations on retailers’ websites and filtering email messages to detect spam.
In these use cases, your analytics results didn’t need to be super accurate in order to be effective. If only 80 percent of the products you recommend to visitors on your website are relevant, that’s pretty acceptable. If your spam filters fail to catch every Nigerian prince email, they still deliver value.
If you examine future-oriented big data use cases, however, you’ll notice that they are much more complex. In addition, the stakes of getting things right are much higher.
For example, smart thermostats that use data analytics to predict your schedule and control your heat accordingly need to be right more than most of the time. When you come home to a cold house because your thermostat did a poor job of predicting when you’d return, it’s more serious than getting an irrelevant product recommendation on a website. Even if this type of mistake happens only a few times a month, it makes your thermostat experience pretty unacceptable.
These challenges become even more serious when they extend to applications like driverless cars, which rely on big data, or power grids. No one wants cars to crash or electricity supplies to fail because the data analytics on which they rely were only 90 or 95 or 99 percent accurate.
Conclusion
Today, the technology doesn’t exist to deliver the ultra-accurate analytics insights that you need for these sorts of use cases. Maybe it will in the future.
For the present, however, it’s worth recognizing the limitations of big data. Big data is a great thing, but it’s not a panacea. It’s better not to use big data at all than to use it and obtain results that cause serious problems for end users.
About the Author
You May Also Like