Why Insight Chose Microsoft Azure Sentinel as Core SIEM Over Splunk
Insight is readying customers for its new managed security service.
March 11, 2020
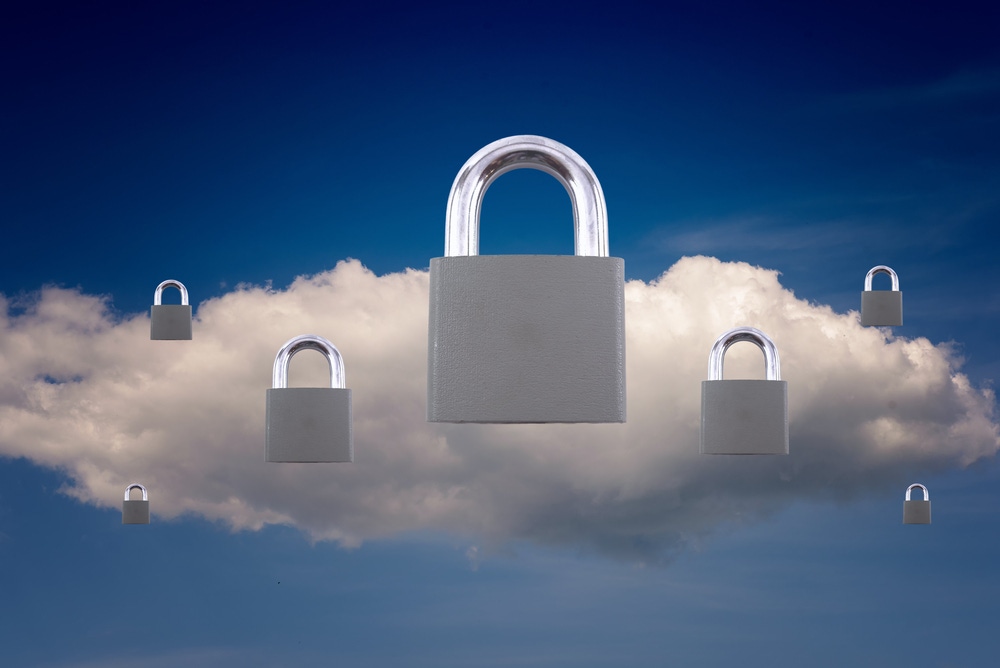
Insight Enterprises, the global systems integration division of Insight Technology Solutions, is among several managed security service providers in the early stages of provisioning customers using Azure Sentinel, Microsoft’s new cloud-native SIEM.
Microsoft introduced Azure Sentinel a year ago as an alternative to traditional on-premises AI-based, threat intelligence solutions such as ArcSight, RSA NetWitness and Splunk. When Azure Sentinel became generally available in late September, Insight Enterprises’ Cloud & Data Center Transformation (CDCD) organization was among the first 20 global partners trained by Microsoft in various stages of adding it to their managed security services.
In addition to Insight, Accenture and its Avanade business, Ascent, DXC Technology, EY Global, Infosys, KPMG, Optiv, PwC, Trustwave and Wipro have said they are building out modernized managed security operations centers (SOCs) hosted with Azure Sentinel.
Microsoft’s Ann Johnson
“We’re seeing more uptake on Azure Sentinel than we could possibly consume right now, which is a fantastic problem to have, which is why we’ve rushed and quickly trained a bunch of partners,” said Ann Johnson, corporate VP for Microsoft’s corporate cybersecurity solutions group, during an interview late last year.
While most of the launch partners offer multiple SIEM options for their SOCs, Insight has decided to base its revamped MSSP with Azure Sentinel as its primary SIEM, according to Richard Diver, a cloud security architect at Insight.
“We’re the only one that I am aware of that is only doing Sentinel; everyone else has something else and then looking to add Sentinel to their list, or they’ll migrate over to Sentinel over time,” Diver said.
Insight also is offering consulting services for customers seeking to migrate their current SOCs to Azure Sentinel.
Azure Sentinel is one of the first of a new class of cloud-native SIEMs that use machine learning at scale to continuously monitor billions of data are native cloud services. Another is Backstory, a security telemetry platform created by Chronicle, incubated from Google parent Alphabet, which last summer became part of Google Cloud.
Amazon launched AWS GuardDuty in 2017, a cloud-scale threat detection offering that monitors and analyzes data sources such as AWS CloudTrail, Amazon VPC Flow Logs and DNS logs. GuardDuty is primarily for AWS workloads, whereas Azure Sentinel can import AWS CloudTrail logs via a connector, Insight’s Diver said. At last month’s RSA Conference, Microsoft announced that customers can import AWS CloudTrail logs at no charge through June 30.
Insight had decided more than a year ago to sunset its ArcSight SIEM and initially was considering running the popular Splunk SIEM as virtual machine instances in AWS, according to Insight’s Diver.
“I stepped in and said that doesn’t make sense economically or technically,” Diver said. “Splunk on prem makes a lot of sense because you’ve got the hardware but trying to run it in AWS or Azure as VMs would cost a fortune. We noticed that a lot of companies that moved to the cloud with VMs in IaaS were coming back because the lift and shift was too expensive.”
Upon learning that Microsoft was developing Azure Sentinel, Diver made the case for it over Splunk, which Insight also sells to enterprises, underscoring the economics of moving Splunk VMs into cloud environments.
“You can’t take something that’s moving petabytes of data from an on-prem environment, and suddenly move to the cloud on a regular basis,” Diver said. “If you’re in the cloud, or going to the cloud, you also don’t want to build Splunk in a VM on Azure or AWS and you don’t want to pull that data back down. Azure Sentinel doesn’t require provisioning of servers, storage, networks, and all the engineering and licensing that goes with building a Splunk environment.”
Diver sees three core scenarios for Azure Sentinel: organizations without …
… any threat detection platform or SIEM; those with on-premises platforms such as Splunk or ArcSight that find it lacks the capabilities for today’s threats; and those who believe Azure Sentinel would be a good compliment to existing Splunk on-premises environments.
Azure Sentinel also makes sense for customers who use other Azure services, Office 365, and even for monitoring data in their AWS or on premises instances, said Diver. Customers pay on a per-gigabyte basis and can store the data for 90 days as part of the base service. They can pay to keep it longer or move it to lower-cost Azure Blob Storage for those who need to hold onto it longer for compliance purpose, Diver added.
“We work really hard at helping customers reduce the cost by making sure they only keep what they need only keep it for as long as they need,” he said.
Microsoft argues, and many experts agree, that cloud-native threat analytics platforms such as Azure Sentinel have the scale that current on-premises SIEMs can’t match when it comes to ingesting data and using AI to detect potential ransomware and malware. Azure Sentinel uses machine learning to help security analysts and data scientists expose legitimate threats, according to Microsoft.
Azure Sentinel’s machine-learning technology, called Fusion, finds threats that “typically fly under the radar by combining low fidelity, ‘yellow’ anomalous activities into high fidelity ‘red’ incidents,” according to engineer Ram Shankar Siva Kumar, data “cowboy” on Microsoft’s Azure data science team.
In a blog posted last month, he explained that Fusion uses machine learning to combine disparate data sources such as network, identity, SaaS and endpoints from Microsoft and from alliance partners.
“Fusion incorporates graph-based machine learning and a probabilistic kill chain to reduce alert fatigue by 90 percent,” he added.
Microsoft revealed last month that telemetry gathered in December from customers that was and fed into Azure Sentinel, identified and graphed, found 50 billion anomalies. Upon applying the probabilistic kill chain, the graph was reduced to 110 subgraphs, followed by another machine-learning process that pulled only 25 incidents actionable by security operations teams.
Read more about:
MSPsAbout the Author
You May Also Like